10 in 5 Olga Dergachyova, Data Scientist
Meet Olga, our Data Scientist at Humblebee with a background in Computer Science and a knack for problem-solving. In this interview, she discusses her journey into Data Science, recommends a great book for enthusiasts, and shares insights on the future of the field.

If you're drawn to the blend of analytical problem-solving and technology, a career in Data Science might be the perfect fit for you! Join us in gaining inspiration from Olga, our Data Scientist at Humblebee, as she sheds light on the significance of data in shaping and developing innovative solutions.
1. Hey Olga, how are you doing today?
Hey! Awesome, thank you.
2. So what do you do at Humblebee?
I’m a Data Scientist.
3. How come you ended up working with Data Science?
I actually have a general Computer Science background. But I did research in the medical field. The project involved lots of data acquisition and processing, as well as Machine Learning (ML). I had to learn a lot on the go and took some additional courses. Later I decided to use all that experience to naturally transition into Data Science (DS).
4. Do you have one good tip for a tool you love, or perhaps a book or resource you highly recommend?
I’m currently finishing a cool book called “Designing Machine Learning Systems” by Chip Huyen. It’s a really good read, especially valuable for people with a research background like mine. Quite often Data Scientists focus on their core job - transforming data, extracting features and training models - but forget about what matters in production - scalability, speed, business metrics, etc. Anyone interested in building performant pipelines for ML applications should read it.
I also like Andrew Ng’s lectures a lot. He has several ML courses, they are a bit old at this point but explain the basics in a very clear and easy-to-understand way.
5. What do you do to make sure you continue to grow as an expert within Data Science?
It’s very hard to stay up-to-date with all the new developments in the field happening at lightning speed. I try to scan the space for ideas and tech that seem fundamental. Something that is going to change how things work and stay for a while, in contrast to buzzy services and models that will be forgotten in a week. I’m subscribed to a couple of newsletters and when they mention something interesting, I go and do deeper research to understand how things work.
6. How do you think Data Science will evolve in the future?
It’s hard to make any confident predictions about the future of DS but I’ll try. I think DS will probably become more integrated with other fields such as healthcare, finance, biology, and more. I believe this interdisciplinary approach can lead to more meaningful insights and solutions. I’d also say some DS aspects will become typical tasks for many people. The most important in that case is to stay humble and curious - benefit from the use of new technologies but avoid an “expert mindset”.
When it comes to us, Data Science specialists, I think more and more basic repetitive tasks will be automated allowing us to focus on high-level analysis and decision-making. At the same time, with an increasing demand for explainable AI models, we will be searching for new reliable models to help us with those high-level tasks as well.
7. If you could use Data Science to solve any everyday problem, what would it be?
I often use my analytical skills in day-to-day life but I don’t think there is anything like that that would make me sit down and write a script to solve the problem using data or AI, haha. I generally think that AI is more and more used for unnecessary things rather than to address important global issues. Personally, I’d be thrilled to work on a project around things that really matter. For example climate change. Solving even small things within the field and seeing progress would make me happy.
8. What part of being a Data Scientist do you find the most enjoyable, and why?
The most enjoyable to me is being faced with a concrete problem for which I need to find a solution. Some problems can be very complex. Periods of failure and impasses are not rare, it was especially true in research. But this makes my brain work and I love it :) It’s also nice to explore new approaches in AI. For example, when a new interesting neural net architecture comes out, I’m always curious to know how it works from a mathematical perspective. I find it intrinsically interesting, don’t know why. Maybe because I like to learn new things and understand how everything works.
9. Do you have any advice for people starting out their career and considering Data Science?
When I started there were fewer things to learn, today my brain would probably explode if I had to start from scratch, haha. On the other hand, there are so many more awesome resources for beginners. My advice is cover the basics. New tools come and go; anyone can quickly learn to use them. Understanding the principles of data wrangling and fundamental ML approaches is much more difficult and will always serve you well. It means understanding statistics, probability theory, linear algebra and calculus. Python is of course a must-have. When it comes to ML models, you don’t need to know how they all work in detail. But you absolutely have to have a high-level understanding of different problem types and how they are usually solved.
10. If you could give a company one piece of advice (from a data scientist perspective)… perhaps a recurring error you have seen from your career… what would that be?
Have a clear strategy for data acquisition and usage. Often companies lack a clear objective for data collection, which leads to a decrease in quality and usability of data. Setting an objective and defining a framework for data acquisition and handling at early stages will help save time and money.
If you’re interested in working at Humblebee then get in touch and we’ll meet up for coffee and a chat.
More stories
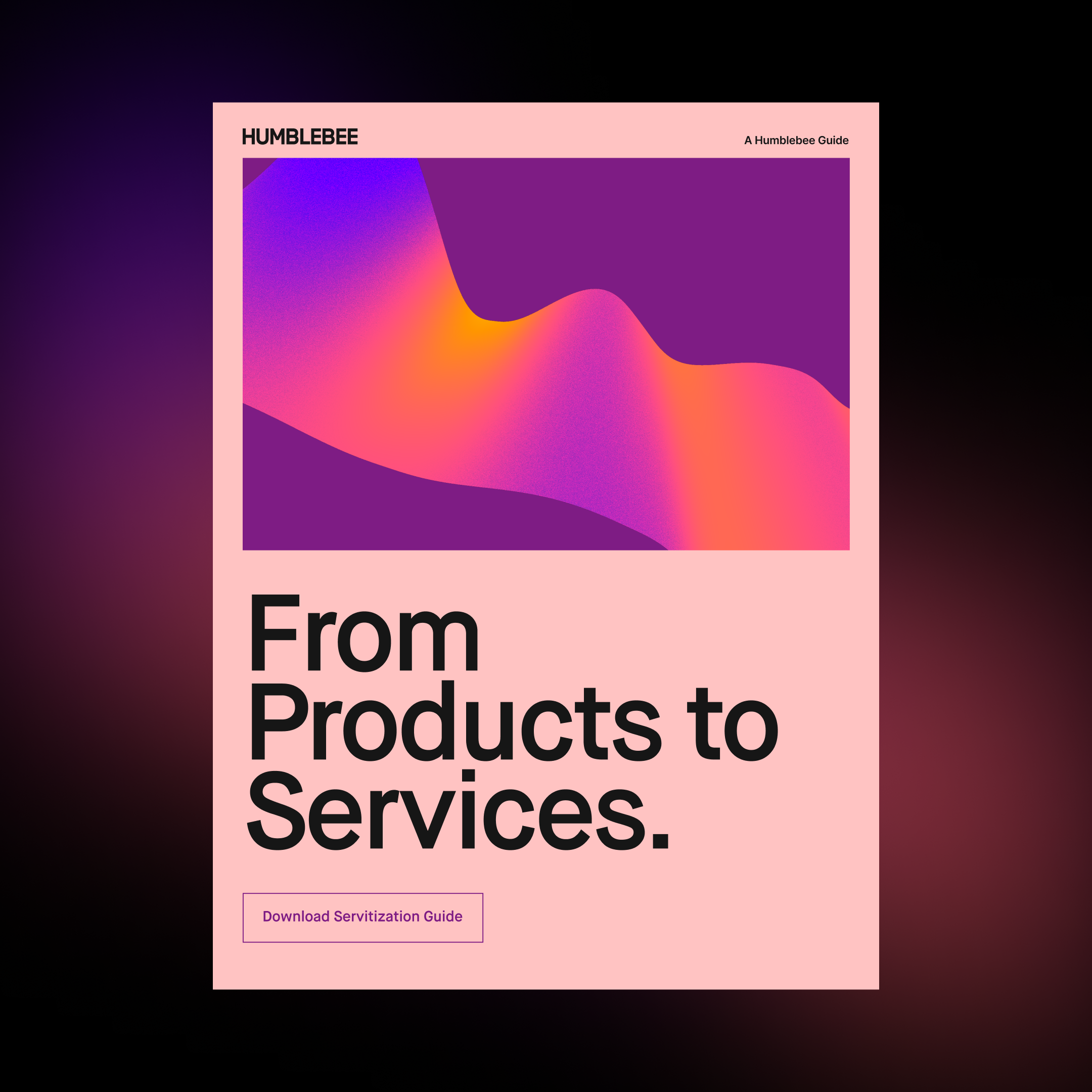
