Navigate the limitations of modern AI and set a successful strategy
AI-powered language models (aka LLMs), such as ChatGPT, have become increasingly popular tools for businesses. As powerful as they may seem, it’s essential to keep in mind their shortcomings to reduce risks related to this technology. I've outlined some shortcomings to watch out for, and some solutions to help you navigate these situations. Enjoy!

Shortcoming 1 - Limited numerical ability
While LLMs can recognise and generate simple numerical information, they lack a deep understanding of mathematics and the ability to perform intricate numerical calculations.
For businesses that rely heavily on data analysis or quantitative decision-making, this limitation can be a significant drawback. Critical tasks like budget forecasting, risk assessment, and performance analysis often require advanced numerical processing that goes beyond the capabilities of LLMs.
Solution: More and more LLMs are able to recognise specific needs and call appropriate functions to perform required tasks. For example, using a calculator to make calculations or Python libraries to analyse and visualise data. Make sure to check in advance if the chosen LLM supports this functionality.
Shortcoming 2 - Incomplete reasoning ability
LLMs do not genuinely understand the text they ingest or generate. They rely on statistical patterns and surface-level associations learned from vast datasets but do not possess cognitive comprehension. Therefore, they might generate plausible-sounding answers that are flawed while failing to assess the quality or appropriateness of the provided arguments. Flawed reasoning may cause significant damage when LLMs are involved in corporate decision-making or strategic planning.
Solution: Ask the model to walk you through its line of reasoning. It has even been shown that this trick helps to generate more factually and logically correct answers. However, always use your own critical thinking to assess the model’s output.
Shortcoming 3 - Lack of self-explainability
LLMs do not generate intermediate representations. They produce a final output without exposing the underlying computations or logic. This lack of self-explainability can be problematic for businesses in sectors such as legal or healthcare that require clear justifications and rationale behind AI-generated recommendations or decisions.
Solution: Many researchers are working on making LLMs more transparent. Until then, try other types of AI-based models and save LLMs for less critical tasks.
Conclusion: Be cautiously ambitious
In conclusion, businesses should exercise caution when integrating LLMs into their operations. While LLMs can offer valuable assistance in various natural language processing tasks, they are not one-size-fits-all solutions.
In many cases, a combination of LLMs with specialised tools, domain-specific models, or human expertise may be necessary to overcome the aforementioned limitations and achieve optimal results.
Our AI experts at Humblebee would be glad to discuss solutions tailored to the specific needs of your particular business.
More stories

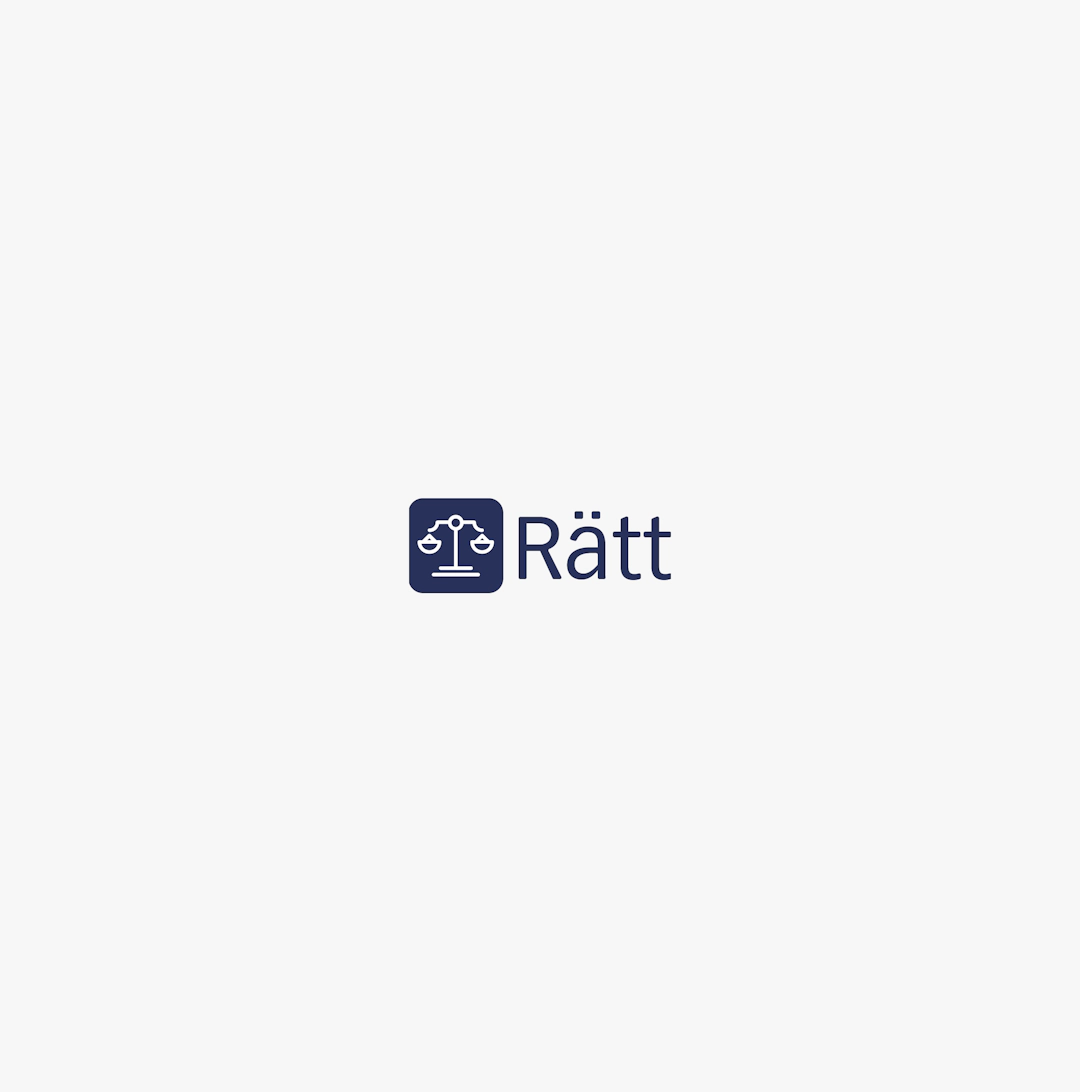